Avoid These 7 Common Mistakes When Implementing Analytics and Machine Learning
Monday, 15 July 2024, 09:00
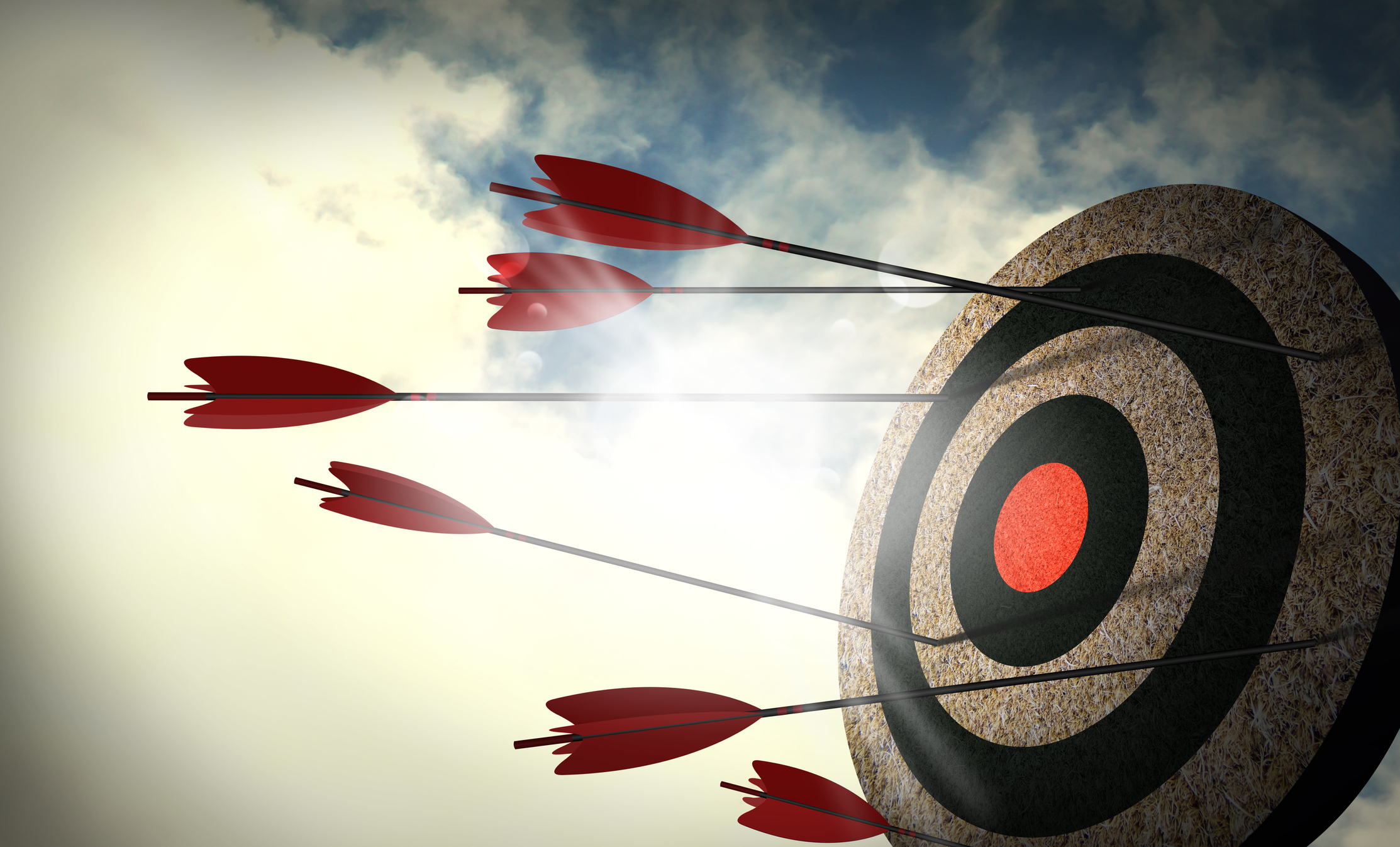
Reasons for Analytics and ML Failures
Implementing analytics and machine learning strategies is crucial for business success, but many projects fall short of expectations. Understanding the common pitfalls can help you avoid similar missteps in your initiatives.
Common Mistakes in Data Projects:
- Failure to Define Clear Objectives: Without a defined goal, projects can easily veer off course, leading to subpar results.
- Ignoring Data Quality: Inaccurate or incomplete data can significantly impact the effectiveness of analytics and ML models.
- Lack of Stakeholder Involvement: Engaging key stakeholders throughout the project is essential for alignment and successful implementation.
These and other factors contribute to the challenges faced by analytics and ML initiatives, making it crucial to address them for improved outcomes.
This article was prepared using information from open sources in accordance with the principles of Ethical Policy. The editorial team is not responsible for absolute accuracy, as it relies on data from the sources referenced.