Bayesian Information Criterion: Unveiling Its Significance in the Realm of Machine Learning
Sunday, 14 July 2024, 12:26
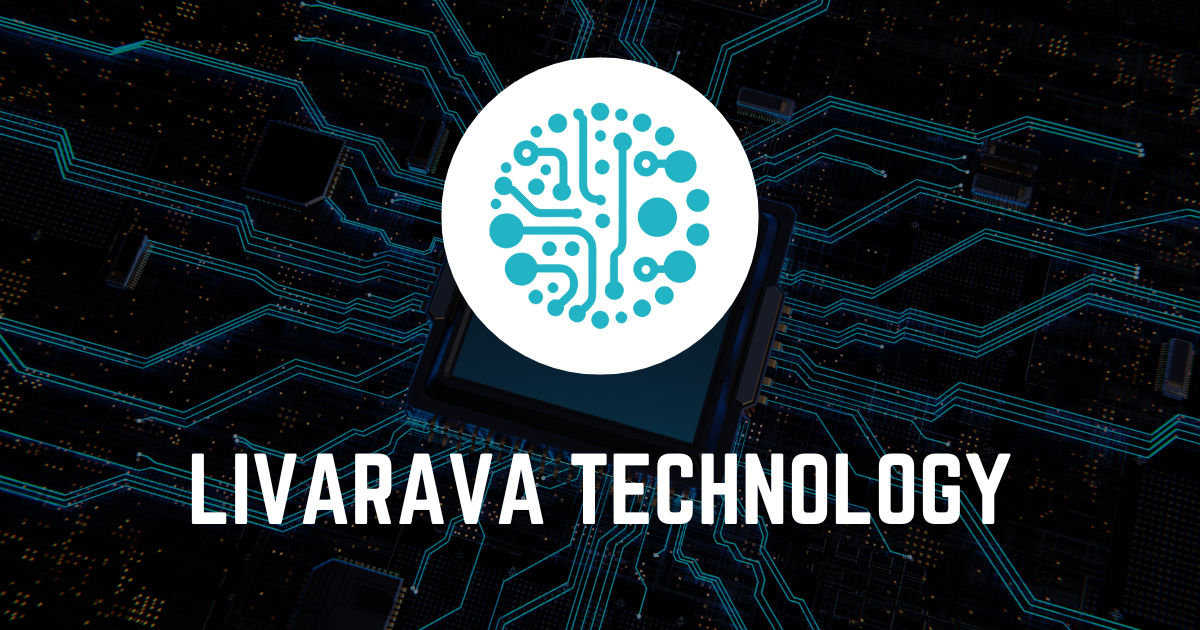
Understanding Bayesian Information Criterion
Discover the critical importance of BIC in model selection
Key Concepts
- Penalization: Balancing model complexity and performance
- Statistical Inference: Utilizing BIC for hypothesis testing
Implications in Machine Learning
- Enhanced Predictive Accuracy: Leveraging BIC for refined modeling
- Efficient Algorithm Development: Streamlining learning processes
- Future Applications: Anticipating advancements in ML with BIC
Conclusively, the exploration of Bayesian Information Criterion in Machine Learning unveils its pivotal role in optimizing model selection and predictive analysis.
This article was prepared using information from open sources in accordance with the principles of Ethical Policy. The editorial team is not responsible for absolute accuracy, as it relies on data from the sources referenced.