Demystifying the Role of Support Vector Machines in Machine Learning
Sunday, 14 July 2024, 12:10
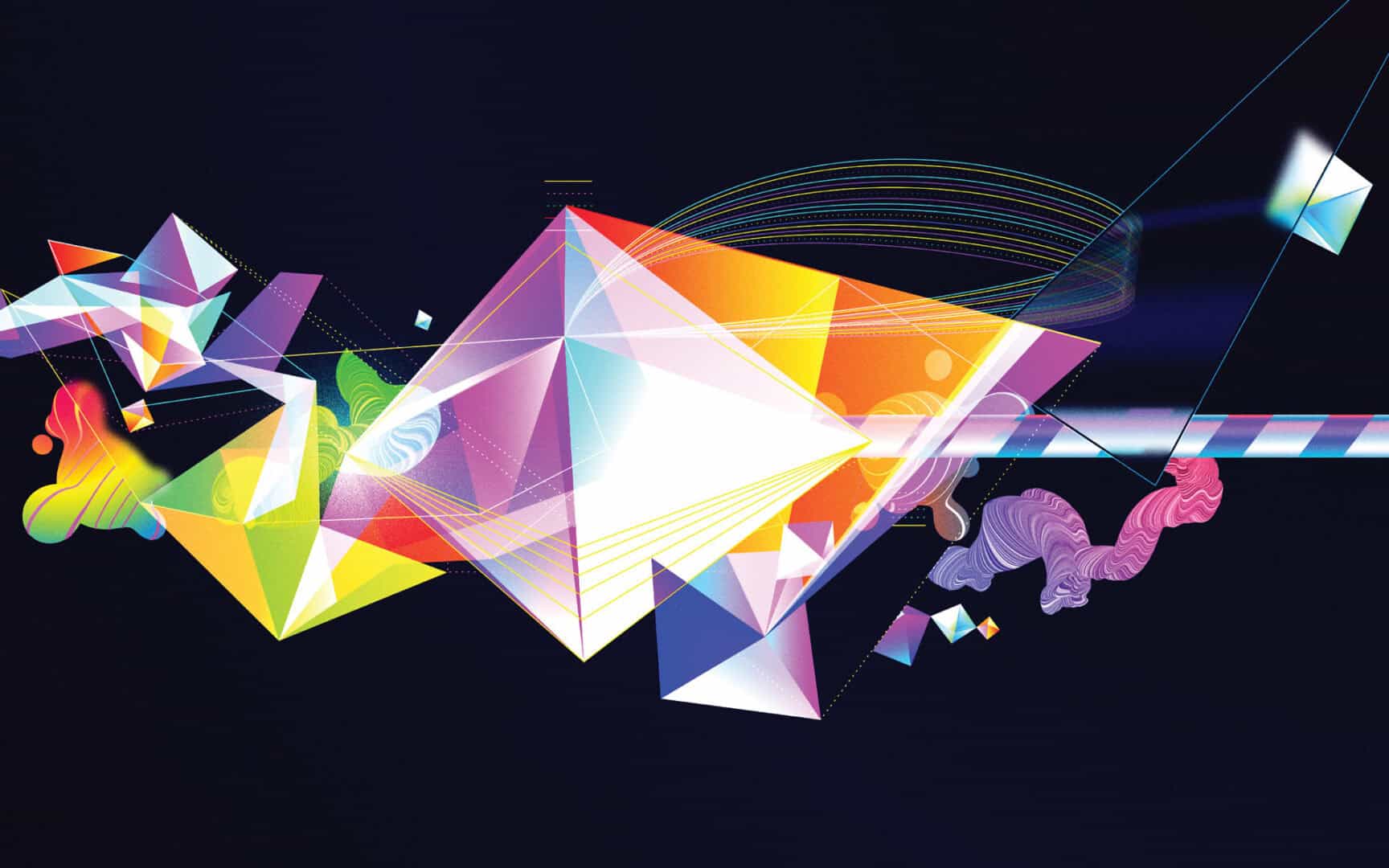
Exploring Support Vector Machines
Support vector machines (SVMs) are powerful tools in machine learning that aim to classify data by finding the optimal hyperplane that separates different classes.
Key Features of SVMs
- Margin Maximization: SVMs seek to maximize the margin between data points, enhancing generalization.
- Kernel Methods: By using kernel functions, SVMs can handle nonlinear data effectively.
Through our detailed analysis, readers will grasp the fundamental concepts behind SVMs and their role in modern machine learning algorithms.
This article was prepared using information from open sources in accordance with the principles of Ethical Policy. The editorial team is not responsible for absolute accuracy, as it relies on data from the sources referenced.