Intervened Reservoir Computing for Enhanced Causal Analysis in Complex Systems
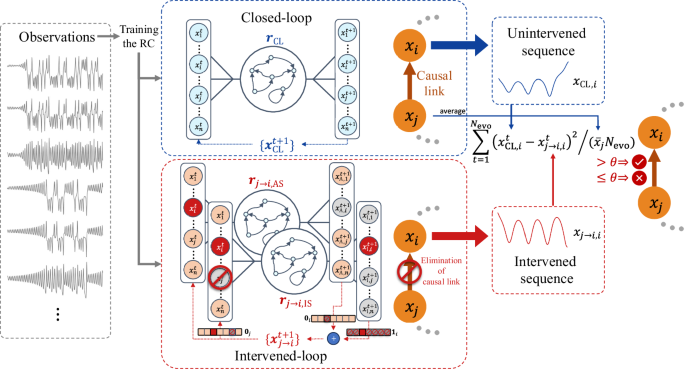
Intervened Reservoir Computing: A Novel Approach for Causal Analysis
An abundance of complex dynamical phenomena exists in nature and human society, requiring sophisticated analytical tools to understand and explain. Causal analysis through observational time series data is essential in comprehending complex systems when controlled experiments are not feasible or ethical.
Unique Approach with IRC
Although data-based causal discovery methods have been widely used, there is still a lack of direct ways aligned with the intuitive definition of causality. The proposed method of intervened reservoir computing (IRC) addresses this gap by constructing a neural network replica of the original system and applying interventions to enable controlled trials.
Accurate Causal Inference
By observing the intervened evolution in the digital twins of the underlying systems, IRC demonstrates its accuracy in inferring causal networks. The study utilizes both simulated and real-world data to showcase the effectiveness of IRC in deciphering causal mechanisms in complex dynamics.
This article was prepared using information from open sources in accordance with the principles of Ethical Policy. The editorial team is not responsible for absolute accuracy, as it relies on data from the sources referenced.