Innovative Machine Learning Predictions for Death Risk in Acute Diquat Poisoning
Friday, 12 July 2024, 09:52
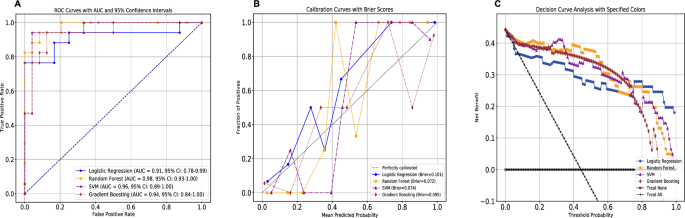
Overview
This study focuses on developing predictive models for assessing death risk in patients with acute diquat (DQ) poisoning.
Methods
- Utilized innovative machine learning techniques such as logistic regression, random forest, SVM, and gradient boosting.
- Evaluated performance in discrimination, calibration, and clinical decision curve analysis.
Results
- Established four reliable machine learning models with AUC values ranging from 0.91 to 0.98.
- SHAP tool integration enhances model transparency and provides explanations for individualized risk prediction.
Conclusion
The developed predictive models offer valuable insights into the death risk assessment in patients with acute DQ poisoning, aiding in clinical decision-making.
This article was prepared using information from open sources in accordance with the principles of Ethical Policy. The editorial team is not responsible for absolute accuracy, as it relies on data from the sources referenced.