Application of Machine Learning Models for Property Prediction in Targeted Protein Degraders
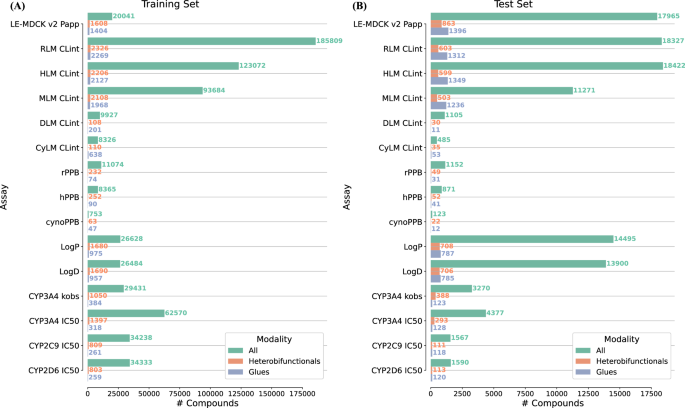
Introduction
Machine learning (ML) systems can model quantitative structure-property relationships (QSPR) using existing experimental data and predict properties for new molecules. With the rise of targeted protein degraders (TPDs), the applicability of ML models for TPD-centric projects is explored.
Key Points
- Performance on TPDs: ML models show comparable performance for TPD predictions in various properties.
- Predictive Errors: Different predictive errors observed for sub-modalities such as glues and heterobifunctionals.
- Transfer Learning: Strategies enhance predictions for heterobifunctionals.
Conclusion
ML-based QSPR models are effective in predicting ADME and physicochemical properties of TPD molecules, supporting their use in drug discovery for TPDs.
This article was prepared using information from open sources in accordance with the principles of Ethical Policy. The editorial team is not responsible for absolute accuracy, as it relies on data from the sources referenced.