Policy Learning with Large World Models: How It Enhances Multi-Task Reinforcement Learning Efficiency
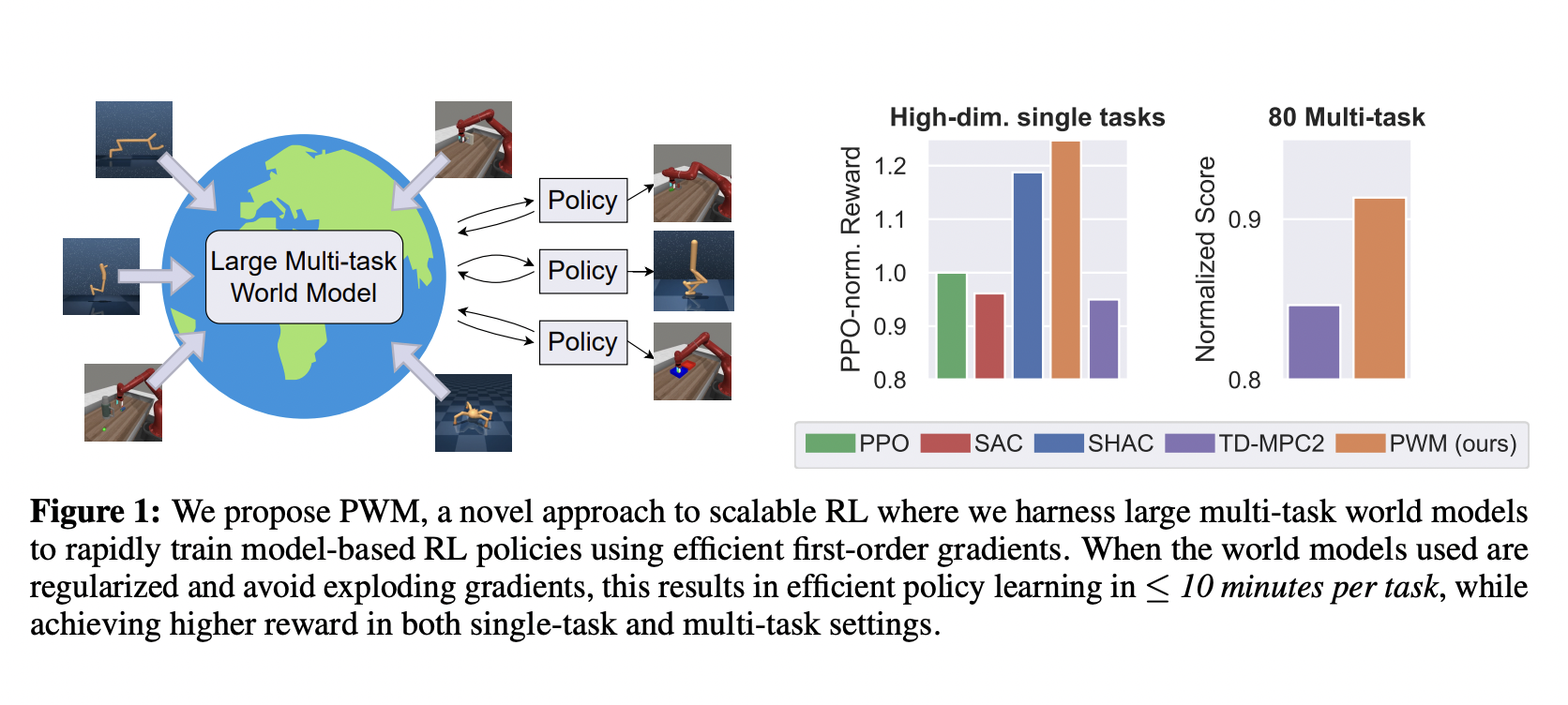
Policy Learning with Large World Models
In the world of AI and machine learning, advancements in multi-task reinforcement learning efficiency are essential for optimal performance.
Enhancing Efficiency
Large world models play a key role in advancing the efficiency of reinforcement learning through policy learning.
Optimal Results
- Optimizing Tasks: Large world models enable optimal results in various tasks simultaneously.
Conclusion
Embracing the latest innovations in policy learning, the use of large world models marks a significant step forward in enhancing the performance of multi-task reinforcement learning.
This article was prepared using information from open sources in accordance with the principles of Ethical Policy. The editorial team is not responsible for absolute accuracy, as it relies on data from the sources referenced.