Revolutionizing Artificial Intelligence with Databricks' Self-Optimizing Algorithms
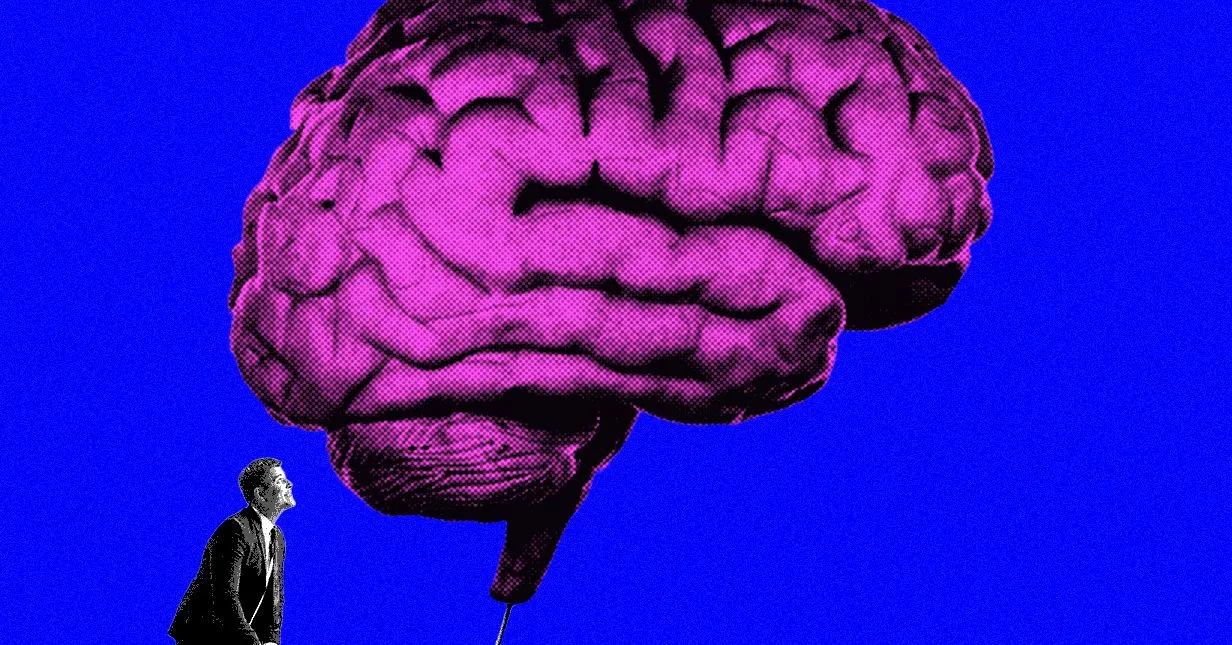
Overview of Databricks' Innovative AI Technology
Artificial intelligence is evolving rapidly, and Databricks has introduced a groundbreaking method for enhancing machine learning algorithms without the need for clean labelled data. Jonathan Frankle, Databricks' chief AI scientist, emphasizes that the primary hurdle many organizations face is data quality. According to Frankle, every company has access to some data but struggles due to its unclean nature.
Addressing Data Quality Challenges
- Companies aim to deploy agents for tasks but lack clean data for fine-tuning.
- Databricks' technique employs reinforcement learning combined with synthetic data.
Key Features of the Databricks Method
Utilizing a method called Test-time Adaptive Optimization (TAO), Databricks showcases how even weak models can enhance performance through a process known as best-of-N. This innovative technique allows for:
- Improvement of language models with minimal labelled data.
- Scalability and effective reinforcement learning application.
Real-World Applications and Impact
Databricks tested the TAO method on FinanceBench, where it surpassed some of the top models like OpenAI's GPT-4. The compelling results demonstrate the potential of AI optimizations in various fields, from finance to healthcare:
- Analysis of key performance indicators in finance.
- Guiding health insurance customers towards relevant information.
Looking Ahead: The Future of AI with Databricks
The future of artificial intelligence is promising as companies explore the applications of TAO to enhance customer models and create more reliable AI agents. With ongoing research and development, Databricks aims to provide innovative solutions that tackle current industry challenges.
This article was prepared using information from open sources in accordance with the principles of Ethical Policy. The editorial team is not responsible for absolute accuracy, as it relies on data from the sources referenced.