Nvidia Nemotron-4 340b: Revolutionizing Synthetic Data Generation in Language Models
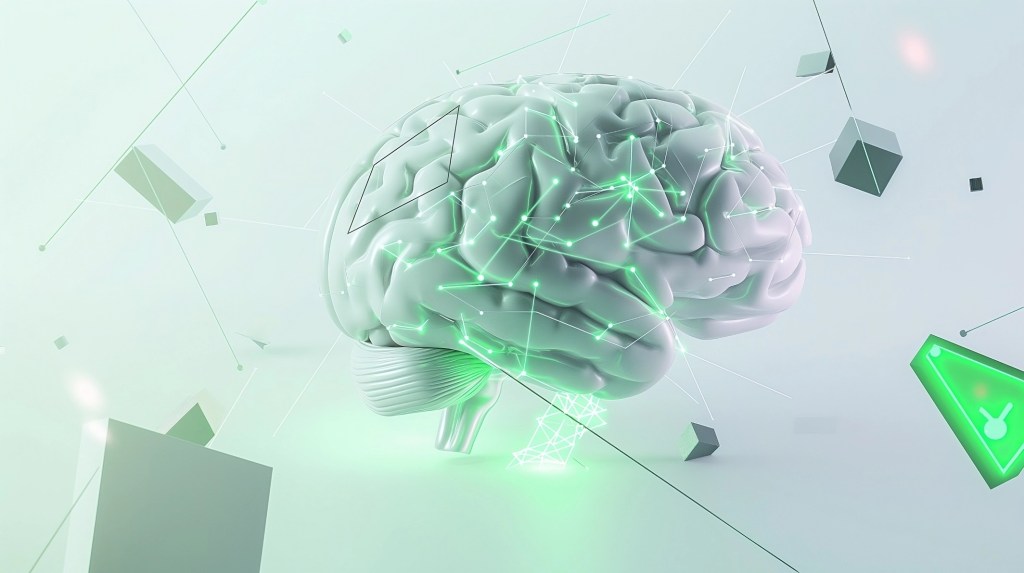
Nvidia has firmly reestablished itself as a trailblazer in artificial intelligence (AI) innovation with the introduction of Nemotron-4 340B, a pioneering family of models designed to revolutionize synthetic data generation for the development of large language models (LLMs). With this development, Nvidia is set to empower various industries to create sophisticated, domain-specific LLMs using cost-effective and efficient synthetic data.
The model, initially known under the alias 'june-chatbot' on LMSys.org, is now officially launched, causing excitement in the AI community.
The Nemotron-4 340B series includes base, instruct, and reward models, forming a holistic pipeline for generating superior synthetic data. With 9 trillion tokens employed in training, a context window of 4,000 tokens, and support for over 50 natural languages and 40 programming languages, Nemotron-4 340B surpasses its competitors, rivalling even GPT-4.
Commercial Viability and Accessibility
One of the standout features of Nemotron-4 340B is its commercially-friendly licensing. According to Senior Deep Learning Research Engineer Somshubra Majumdar, “The license is commercially viable. Yeah, you can use this to generate all the data you want.” Nvidia's commitment to accessible AI tools ensures that even small-to-medium enterprises can leverage comprehensive LLMs without prohibitive costs.
Powerful Performance and Broad Applications
Nvidia’s new model demonstrates unparalleled performance and versatility in synthetic data generation, attributed to its optimized designs for generative AI training. The Nemotron-4 340B family, especially the Reward model, has already claimed the top position at the Hugging Face RewardBench leaderboard, exemplifying its capabilities.
Additional advantages include extensive customization and fine-tuning options. Researchers can enhance the Base model with their data, tailoring it to specific industry needs, whether for healthcare advancements, financial risk assessments, or retail supply chain optimizations.
Nemotron-4 340B's Impact Across Industries
The potential benefits of the Nemotron-4 340B are immense across several sectors:
- Healthcare: Generate synthetic data for breakthroughs in drug discovery, personalized medicine, and medical imaging.
- Finance: Train LLMs for improved fraud detection, risk assessment, and enhanced customer service.
- Retail and Manufacturing: Optimize predictive maintenance, supply chains, and personalize customer interactions.
Ethical and Security Considerations
As synthetic data usage grows, the conversation around data privacy and ethical implications becomes critical. Businesses need robust safeguards to protect sensitive information and ethical considerations to avoid biases and inaccuracies.
Despite these challenges, the AI community's response to the Nemotron-4 340B has been overwhelmingly positive. Initial user feedback highlights impressive performance and domain-specific knowledge, lauding Nvidia’s latest AI breakthrough.
FAQ
What is the Nvidia Nemotron-4 340B?
Nvidia Nemotron-4 340B is a new family of models designed to revolutionize synthetic data generation for large language models, supporting over 50 natural languages and 40 programming languages.
How does the Nemotron-4 340B model support various industries?
The Nemotron-4 340B supports industries like healthcare, finance, and retail by enabling the generation of high-quality synthetic data tailored for specific applications, resulting in advancements in drug discovery, financial risk assessments, and supply chain optimizations.
What sets the Nemotron-4 340B apart from other models?
The Nemotron-4 340B is distinguished by its extensive training on 9 trillion tokens, commercially-friendly licensing, and support for a wide range of languages. It also offers customization and fine-tuning options for domain-specific model development.