Research on AI Deep Learning Identifies Key Quantization Limits
Sunday, 17 November 2024, 15:00
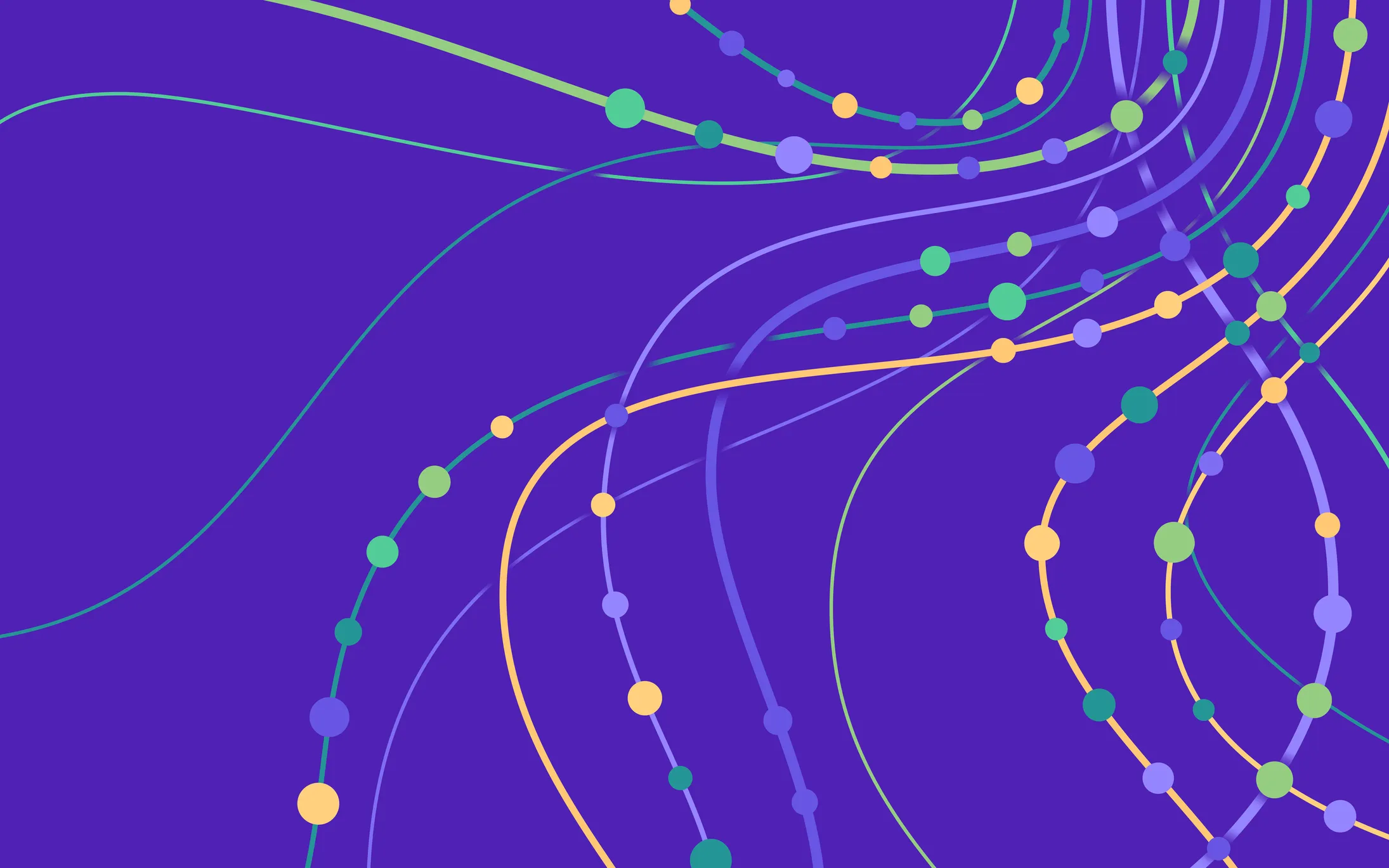
Research Unveils Limitations in AI Deep Learning Quantization
Recent research emphasizes the need to reassess the widely applied quantization methods in AI and deep learning. While quantization improves model efficiency by reducing bit depth, it also introduces potential pitfalls.
- Efficiency vs. Accuracy: Lowering bit rates simplifies computation, but may reduce accuracy.
- Industry Implications: Companies heavily investing in quantization must stay informed about emerging challenges.
- Future Research Directions: Ongoing studies are essential to develop standards that balance efficiency with performance.
Key Findings from the Study
- Quantization techniques have inherent trade-offs.
- Understanding the limitations is crucial for reliable deployments.
- This research could redefine strategies in AI development moving forward.
This article was prepared using information from open sources in accordance with the principles of Ethical Policy. The editorial team is not responsible for absolute accuracy, as it relies on data from the sources referenced.