Artificial Intelligence in Space Missions: Insights from Michele Faragalli of Mission Control
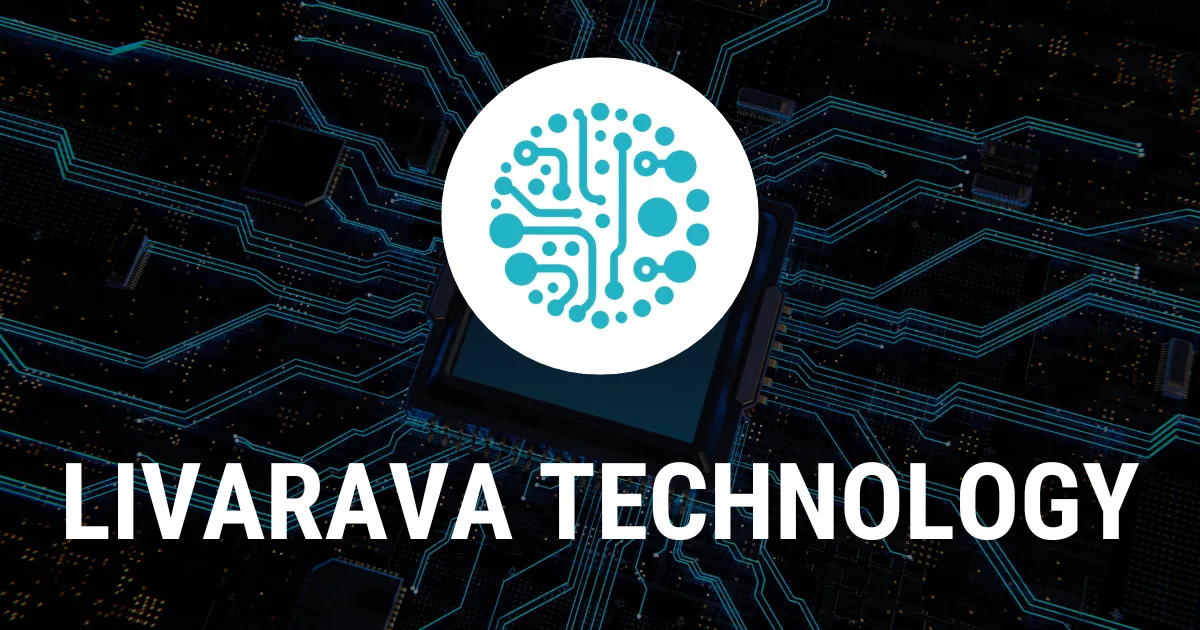
Artificial Intelligence in Space Missions: The Unique Challenges
Deploying artificial intelligence in space is fraught with complexities unlike those faced on Earth. Michele Faragalli, CTO of Mission Control, explains how unique environmental factors affect the deployment of deep learning models. This is particularly evident during a collaboration with Spire, launching a demo mission in 2025.
The Need for Advanced Machine Learning Models
Spacecraft require advanced machine learning to tackle high latency and limited bandwidth issues. Advanced models allow for immediate data processing, enhancing response times for critical events like natural disasters. The mission aims to prove that real-time onboard analysis can lead to quicker decisions.
Training AI in Space Conditions
While training models on Earth is feasible, operating conditions in space pose unique challenges. Artificial intelligence must adapt to unpredictable environments. Michele elaborates on the necessity of simulation with synthetic data for extraterrestrial operations.
Handling the Uncertainty of Spaceflight
Mission Control's collaboration is focused on developing methodologies for machine learning operations, or MLOPs, applicable to unique space conditions. This endeavor not only aims to improve model reliability but also the overall adaptability of spacecraft.
Lack of Certification Standards
An ongoing issue in space missions is the absence of certification standards pertinent to deep learning technologies. Michele highlights the uncertainty surrounding statistical performance and the inherent difficulties in validating these algorithms for critical space applications.
This article was prepared using information from open sources in accordance with the principles of Ethical Policy. The editorial team is not responsible for absolute accuracy, as it relies on data from the sources referenced.