Revolutionizing Generative AI with Thought Preference Optimization for Smarter Outcomes
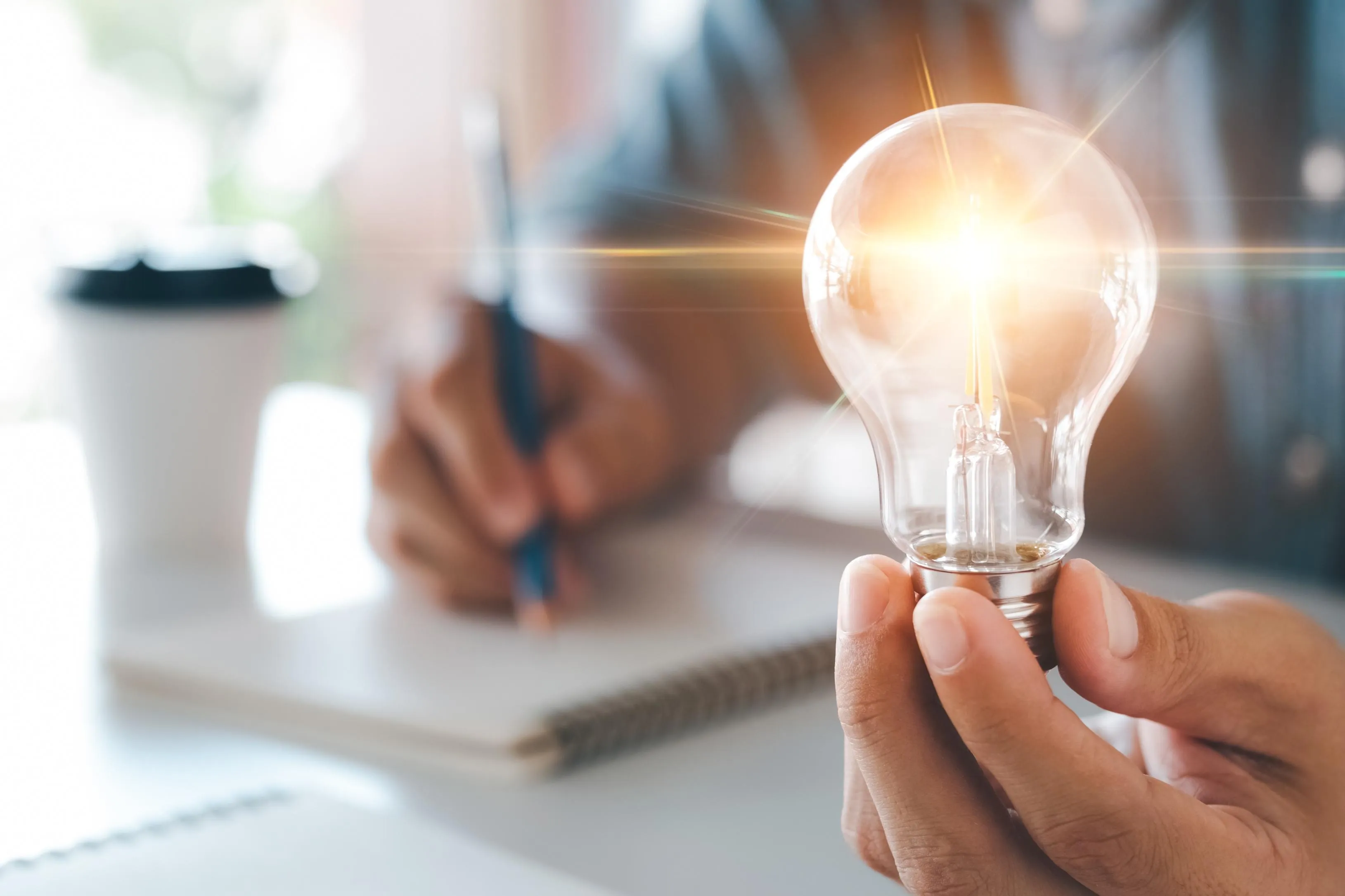
Discovering the Advances in AI
Generative AI is on the rise, and recent improvements in logic-driven problem-solving techniques, particularly through Thought Preference Optimization (TPO), are changing the landscape. Used in combination with large language models (LLM), this innovative method offers smarter, more efficient outcomes. Meta, with collaborations from UC Berkeley and NYU, is at the forefront of this technological evolution.
Key Insights into Thought Preference Optimization
- Understanding the Mechanics: TPO enhances generative AI by structuring problem-solving processes.
- The Influence of Chain of Thought (COT): This methodology further refines AI's decision-making capabilities.
- Future Implications: Such advances could redefine user interaction with generative AI systems.
Embracing these innovations allows developers and researchers to push the boundaries of what AI can accomplish. For those intrigued by the rapid progress in AI technologies, staying informed on these developments is essential.
This article was prepared using information from open sources in accordance with the principles of Ethical Policy. The editorial team is not responsible for absolute accuracy, as it relies on data from the sources referenced.