Uncovering Limitations in Long-Context LLMs with DeepMind's Michelangelo Benchmark
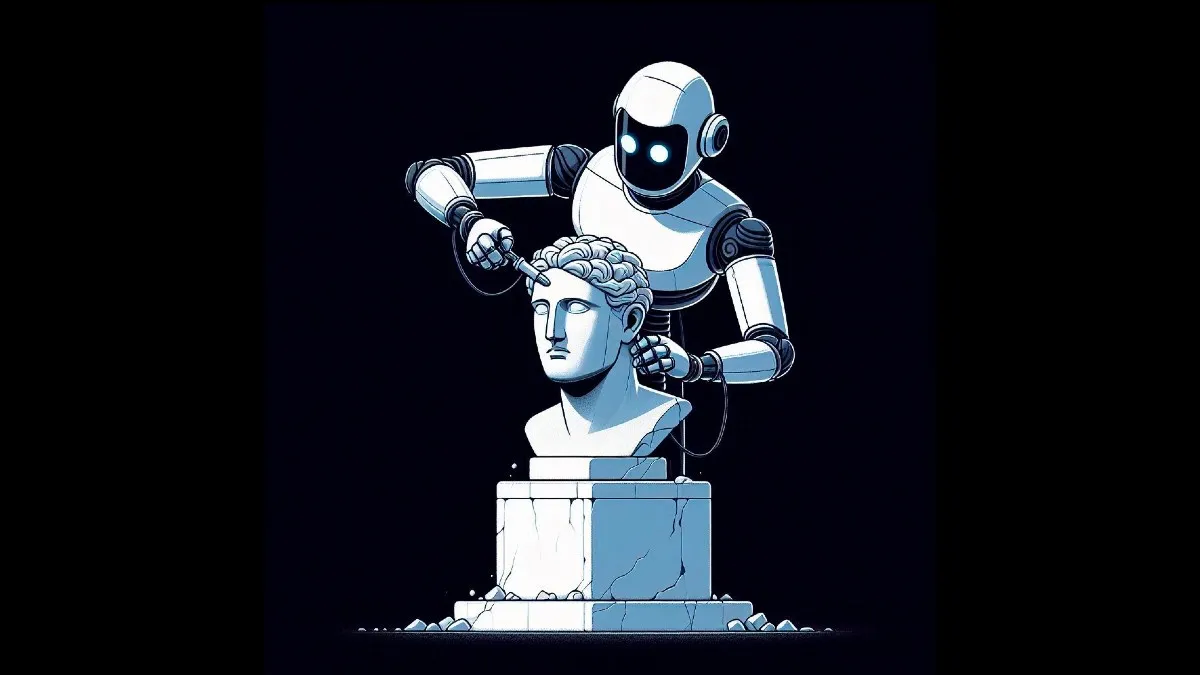
Introduction to DeepMind's Michelangelo Benchmark
DeepMind has developed the Michelangelo benchmark to evaluate the performance of long-context LLMs. This benchmark is crucial in highlighting key areas where these models excel and where they fall short.
Key Findings
- LLMs can efficiently retrieve information from their context windows but face challenges in reasoning.
- DeepMind’s tests emphasize the limitations in logical processing when the context becomes extensive.
- The need for upgrades in model architecture to improve reasoning capabilities is necessary.
Future Directions
The outcomes from this benchmark pave the way for future research aimed at enhancing the reasoning abilities of LLMs. Promoting discussions within the community about better architectures will be essential.
This article was prepared using information from open sources in accordance with the principles of Ethical Policy. The editorial team is not responsible for absolute accuracy, as it relies on data from the sources referenced.