Deep Learning-powered Speckle Imaging for Fast Powder Size Detection
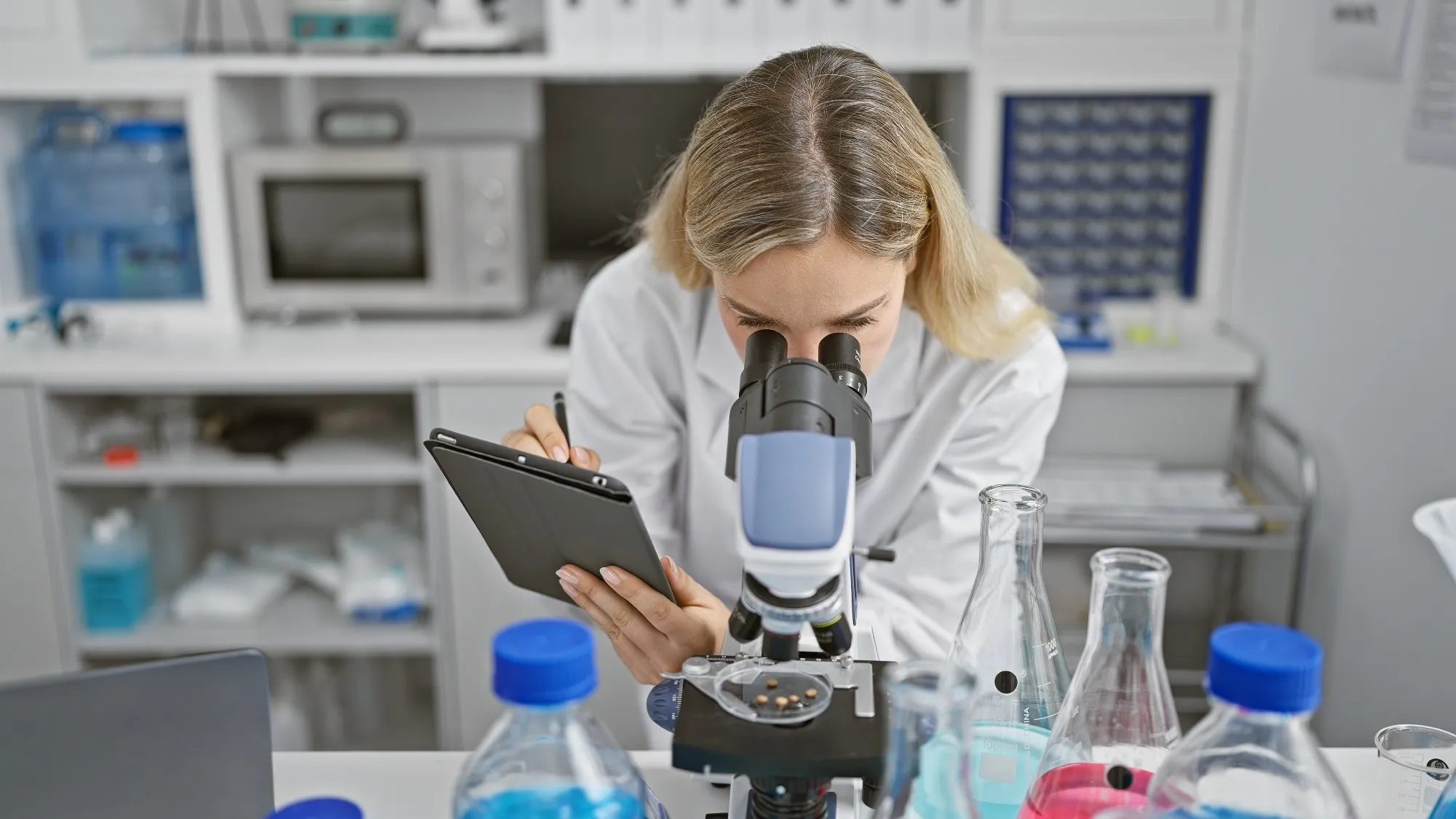
Deep Learning and Speckle Imaging: A New Paradigm
In recent years, Deep Learning has revolutionized numerous fields, and powder size detection is no exception. Traditional methods often require complex setups and extensive sample processing; however, the innovative approach of **Deep Learning-based speckle imaging** simplifies this. It utilizes a single speckle image to assess powder size distribution with remarkable accuracy.
Enhancing Measurement Efficiency
The integration of pupil function engineering allows the method to optimize the light patterns used during imaging, enhancing the measurement process. By employing sophisticated deep learning models, this technique reduces the time needed for analysis, making it a valuable tool for industries reliant on precision measurement.
Applications and Implications
- Pharmaceutical manufacturing
- Material science development
- Quality control in powdered products
Overall, the **Deep Learning-based speckle imaging** technique marks a significant advancement in the field, potentially transforming powder size analysis.
This article was prepared using information from open sources in accordance with the principles of Ethical Policy. The editorial team is not responsible for absolute accuracy, as it relies on data from the sources referenced.