Machine Learning and AI: Navigating Cost Efficiency Challenges in Cloud Computing
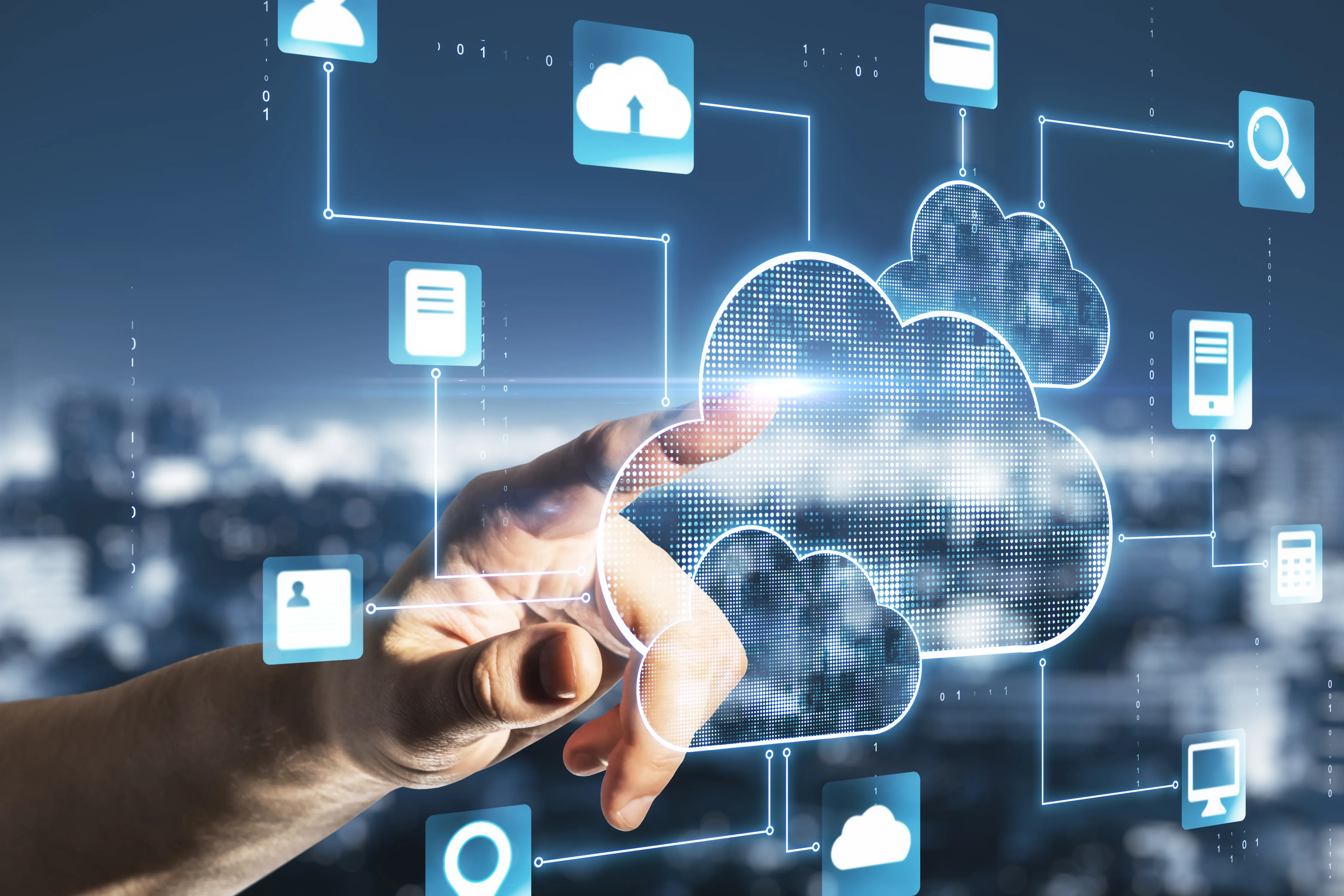
Machine Learning and AI: Navigating Cost Efficiency Challenges in Cloud Computing
The integration of machine learning and AI is resulting in significant pressures on operational budgets for companies leveraging cloud infrastructures. The exponential growth of data recorded and the relentless requirement for computational power to train various AI models mean that organizations face mounting costs. Companies must find ways to balance the demand for innovation in the cloud with the imperative of cost management.
AI's Impact on Cloud Costs - Managing the Challenge
- Machine learning methods require substantial computational power and vast data storage.
- AI workloads often depend on specialized, expensive hardware that exceeds the cost of standard resources.
- Fluctuating workloads complicate the prediction of computing needs, further straining budgets.
Despite these challenges, AI and machine learning can also provide solutions to their own cost challenges. By automating processes and optimizing resource usage, companies can manage costs more effectively.
AI-Powered Solutions for Cost Management
- Optimization Algorithms: These algorithms can automatically adjust resource levels based on usage patterns, helping to prevent overspending.
- Predictive Models: By analyzing historical data, predictive models can prepare businesses for peak usage periods, reducing costs during low-demand times.
- Real-Time Monitoring: AI-driven FinOps tools enable organizations to track cloud resource consumption in real time, unveiling cost-saving opportunities.
Through these innovative approaches, companies can leverage the strengths of AI to achieve sustainable success in their operations, optimizing both cost and performance in a competitive landscape.
This article was prepared using information from open sources in accordance with the principles of Ethical Policy. The editorial team is not responsible for absolute accuracy, as it relies on data from the sources referenced.