Forecasting Calderas and Their Hazards with Advanced Machine Learning Techniques
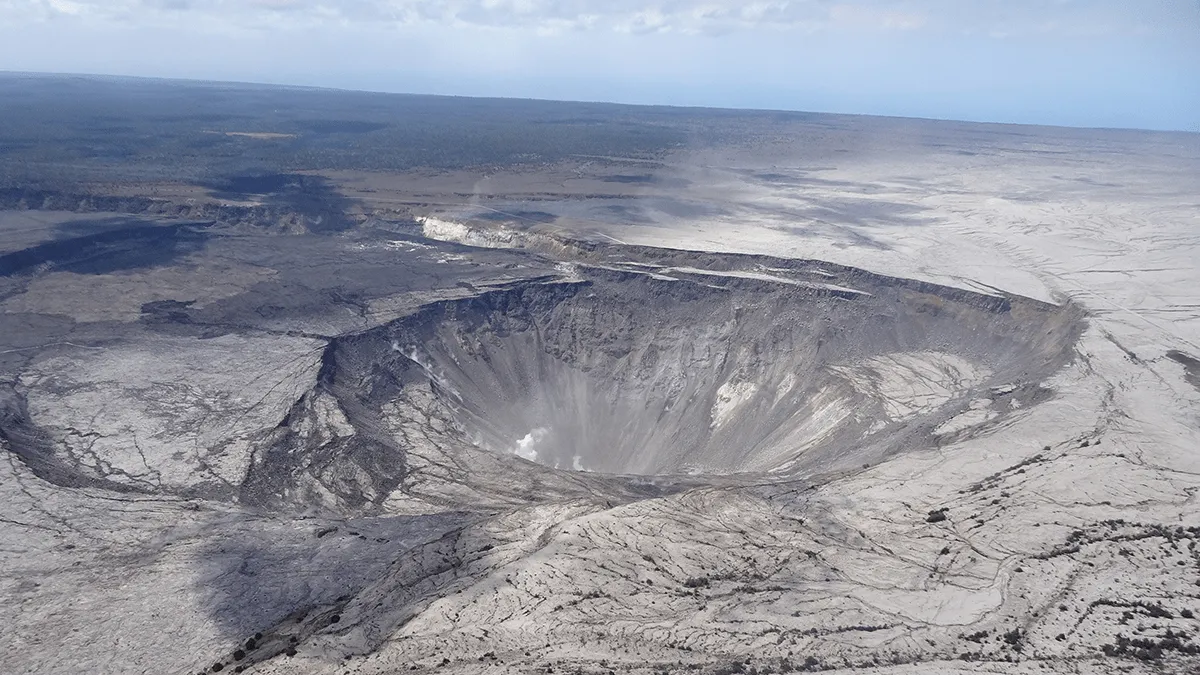
Understanding Calderas: A Deep Dive
Calderas are huge volcanic depressions formed when a volcano erupts and collapses inward. These formations can pose significant hazards and disasters if not properly monitored. In recent years, advancements in machine learning and AI have revolutionized our approach to earth science by offering new forecasting capabilities.
Machine Learning & AI in Volcano Forecasting
Utilizing data from the 2018 Kilauea volcano eruption in Hawaii, researchers developed a deep learning model that effectively predicts caldera collapses. This model leverages geophysical data to identify patterns and deliver insights that were previously unattainable through traditional methods.
- Key Findings:
- Enhanced accuracy in predicting caldera collapses
- Utilizes vast datasets for improved modeling
- Integrates seismology with modern AI techniques
Future Implications
As we advance our research in hazards and disasters, these emerging technologies can lead to better preparedness and risk mitigation measures for caldera-related volcanic activity.
This article was prepared using information from open sources in accordance with the principles of Ethical Policy. The editorial team is not responsible for absolute accuracy, as it relies on data from the sources referenced.