Exploring the 'Arrow of Time' Effect in Large Language Models
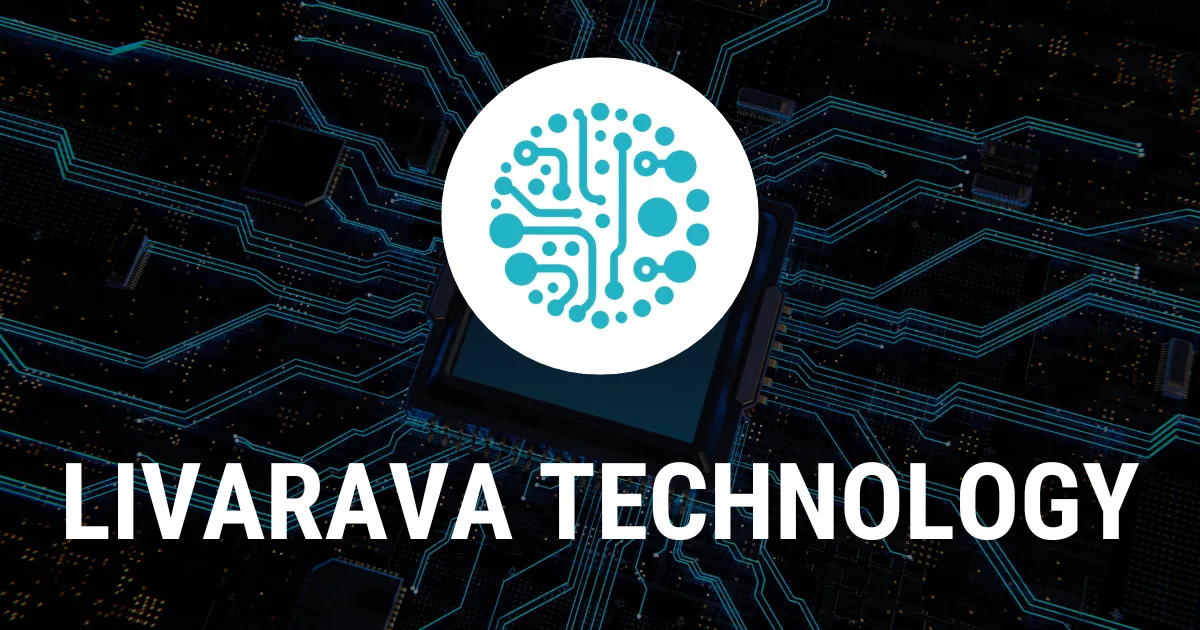
Understanding the 'Arrow of Time' Effect
Recent studies have highlighted the intriguing 'Arrow of Time' effect in large language models (LLMs), particularly in their ability to predict subsequent words in a sentence more effectively than the preceding ones. This innovative behavior challenges conventional approaches to AI. Researchers suggest that exploring this effect can significantly enhance the performance of models such as GPT-4.
Implications for AI Language Processing
As we delve deeper into how LLMs function, understanding the implications of this effect is crucial. It not only augments the efficiency of predictions but also opens avenues for developing more sophisticated algorithms that capitalize on temporal sequences in language processing techniques.
- Focus on future prediction workflows
- Analysis of changes in model training
- Comparison of traditional versus LLM approaches
This article was prepared using information from open sources in accordance with the principles of Ethical Policy. The editorial team is not responsible for absolute accuracy, as it relies on data from the sources referenced.