Zitnik Lab's AI Revolutionizes Drug Repurposing for Rare Diseases
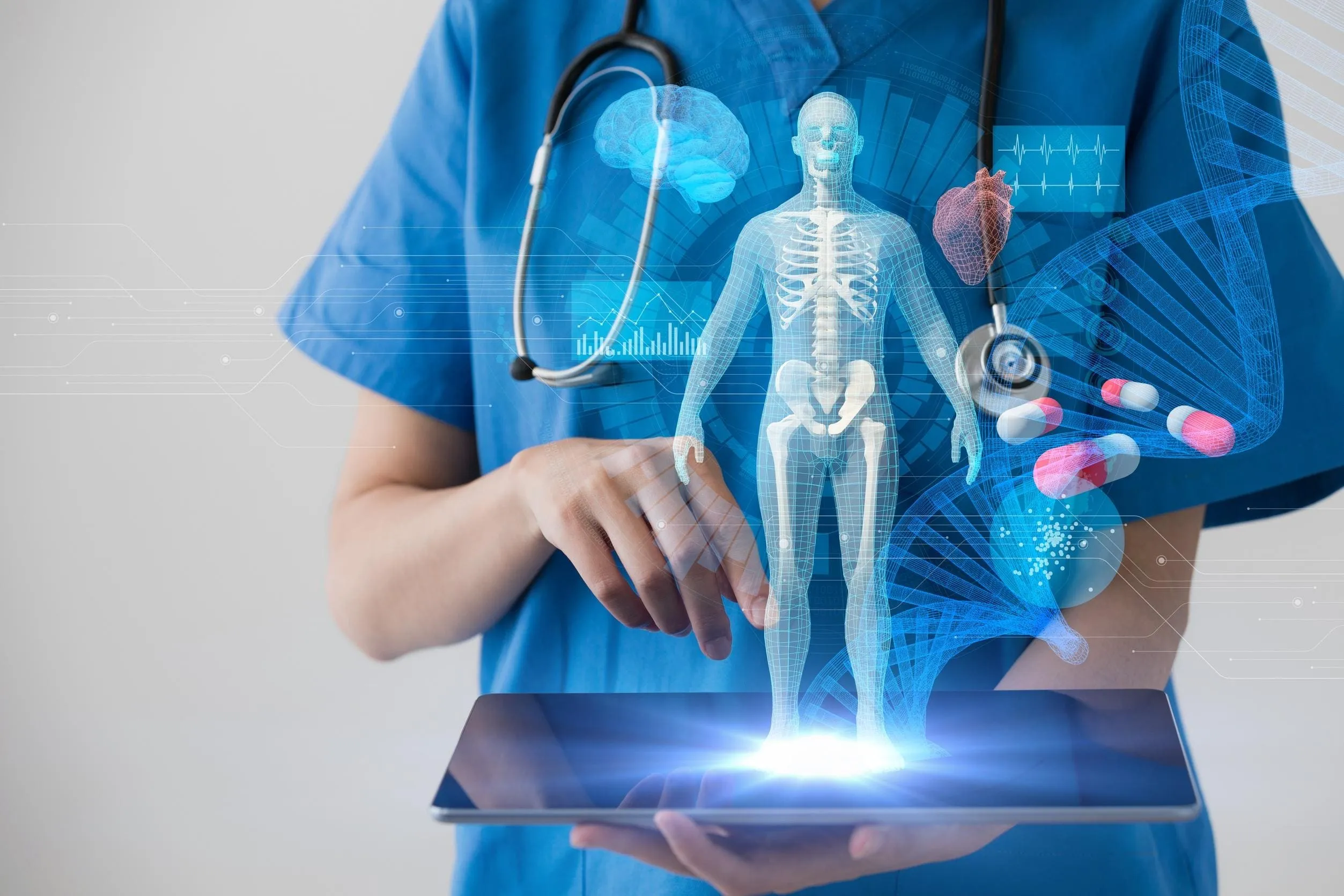
Revolutionizing Drug Discovery with AI
Zitnik Lab's latest innovation showcases how artificial intelligence, particularly the TxGNN model, is revolutionizing drug discovery and repurposing for rare diseases. This groundbreaking initiative marks a pivotal advancement in medical technology, allowing for efficient identification of suitable drug candidates.
Key Features of TxGNN
- Zero Shot Inference: This unique approach allows the AI model to make predictions for rare diseases without prior examples.
- Increased Efficiency: TxGNN accelerates the identification process, paving the way for faster treatment solutions.
- Broad Applicability: Designed to cover a large array of rare diseases, offering hope for numerous patients.
The Future of Drug Discovery
As we continue to witness the fusion of technology and healthcare, tools like TxGNN exemplify how innovative approaches are essential to solving pressing medical challenges. The implications of this AI-driven model extend beyond traditional methods, representing a promising future in drug discovery.
This article was prepared using information from open sources in accordance with the principles of Ethical Policy. The editorial team is not responsible for absolute accuracy, as it relies on data from the sources referenced.