ΔHbT1-T0 and Machine Learning for Identifying HPO in Dyspnoea Patients
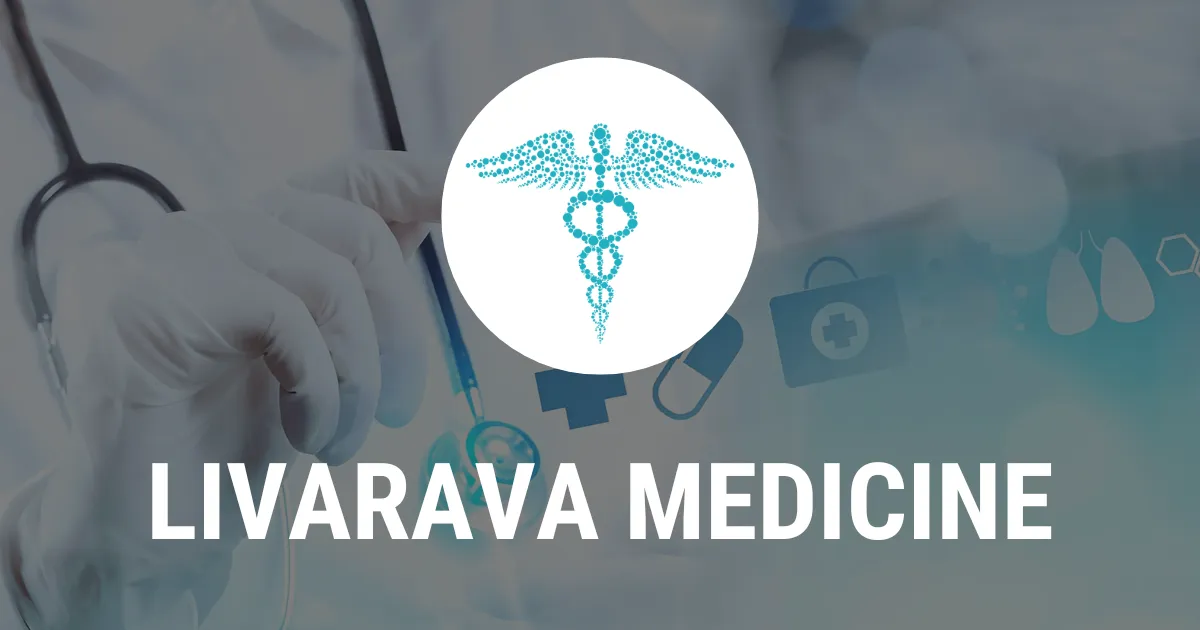
Understanding ΔHbT1-T0 in Dyspnoea
The use of ΔHbT1-T0 is becoming increasingly significant in clinical settings, particularly for assessing patients presenting with acute dyspnoea. This physiological marker provides crucial information regarding a patient's oxygenation status.
The Role of Machine Learning
Machine learning algorithms are transforming how healthcare professionals interpret ΔHbT1-T0 readings. By analyzing vast datasets, these tools can swiftly predict Hypoxia-Related Outcomes (HPO), leading to enhanced diagnostic accuracy.
Implications for Emergency Departments
- The integration of machine learning into clinical practice represents a significant leap in medical technology.
- Emergency departments can benefit by improving turnaround times for patient assessments.
- This approach could reduce hospital stays and optimize resource allocation.
For further details on the findings and methodologies, please visit the original source.
This article was prepared using information from open sources in accordance with the principles of Ethical Policy. The editorial team is not responsible for absolute accuracy, as it relies on data from the sources referenced.