Raman Spectra Classification for Crystal Conditions Using Machine Learning
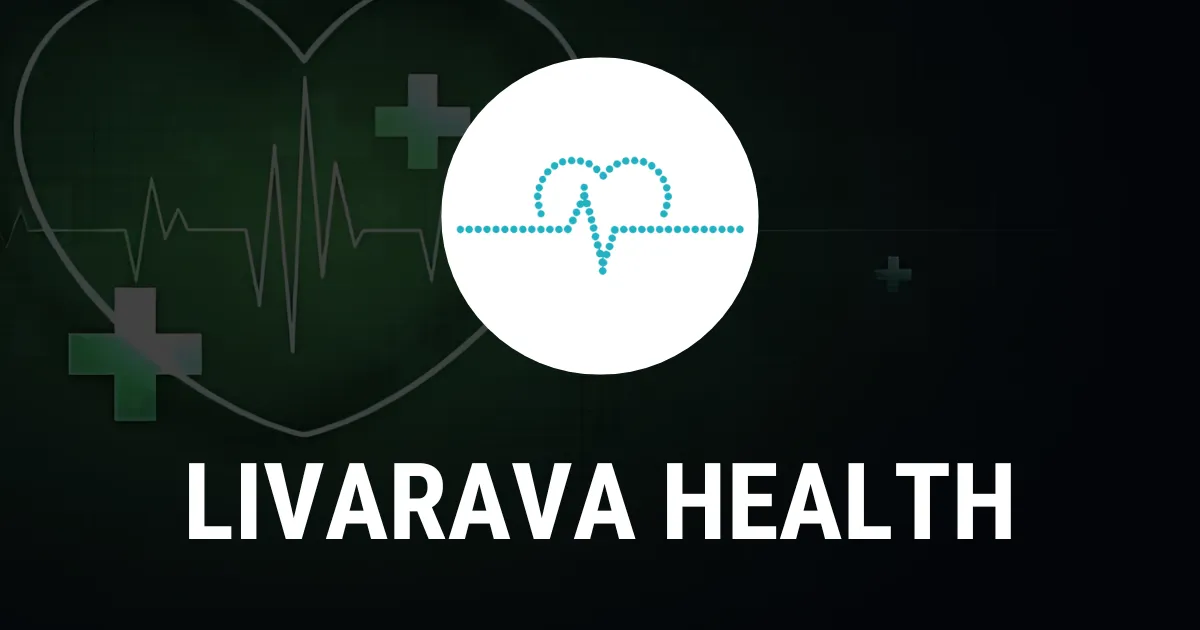
Advancements in Health Diagnostics
Machine learning is transforming the way we approach health diagnostics. In particular, Raman spectra classification is emerging as a valuable tool for identifying crystal conditions such as gout and calcium pyrophosphate deposition disease. Traditional methods may involve subjective interpretation, but with the power of machine learning, the process can become much more objective and precise.
Understanding Raman Spectra
Raman spectra refers to the inelastic scattering of light that provides information about molecular vibrations. This technique can be particularly useful in a point-of-care setting for swiftly diagnosing conditions that involve crystal deposits within the body.
- Improved accuracy
- Faster diagnosis
- Better patient management
Benefits of Machine Learning in Healthcare
- Enhanced diagnostic capabilities
- Real-time data processing
- Potential for widespread application
These advancements signal a new era in health technology where machine learning plays a crucial role in diagnostics, especially concerning crystal conditions. As research continues to develop in this area, we can anticipate improvements in both diagnosis and treatment outcomes for patients.
Disclaimer: The information provided on this site is for informational purposes only and is not intended as medical advice. We are not responsible for any actions taken based on the content of this site. Always consult a qualified healthcare provider for medical advice, diagnosis, and treatment. We source our news from reputable sources and provide links to the original articles. We do not endorse or assume responsibility for the accuracy of the information contained in external sources.
This article was prepared using information from open sources in accordance with the principles of Ethical Policy. The editorial team is not responsible for absolute accuracy, as it relies on data from the sources referenced.