Machine Learning and AI: Key Drivers of Cost Efficiency Challenges
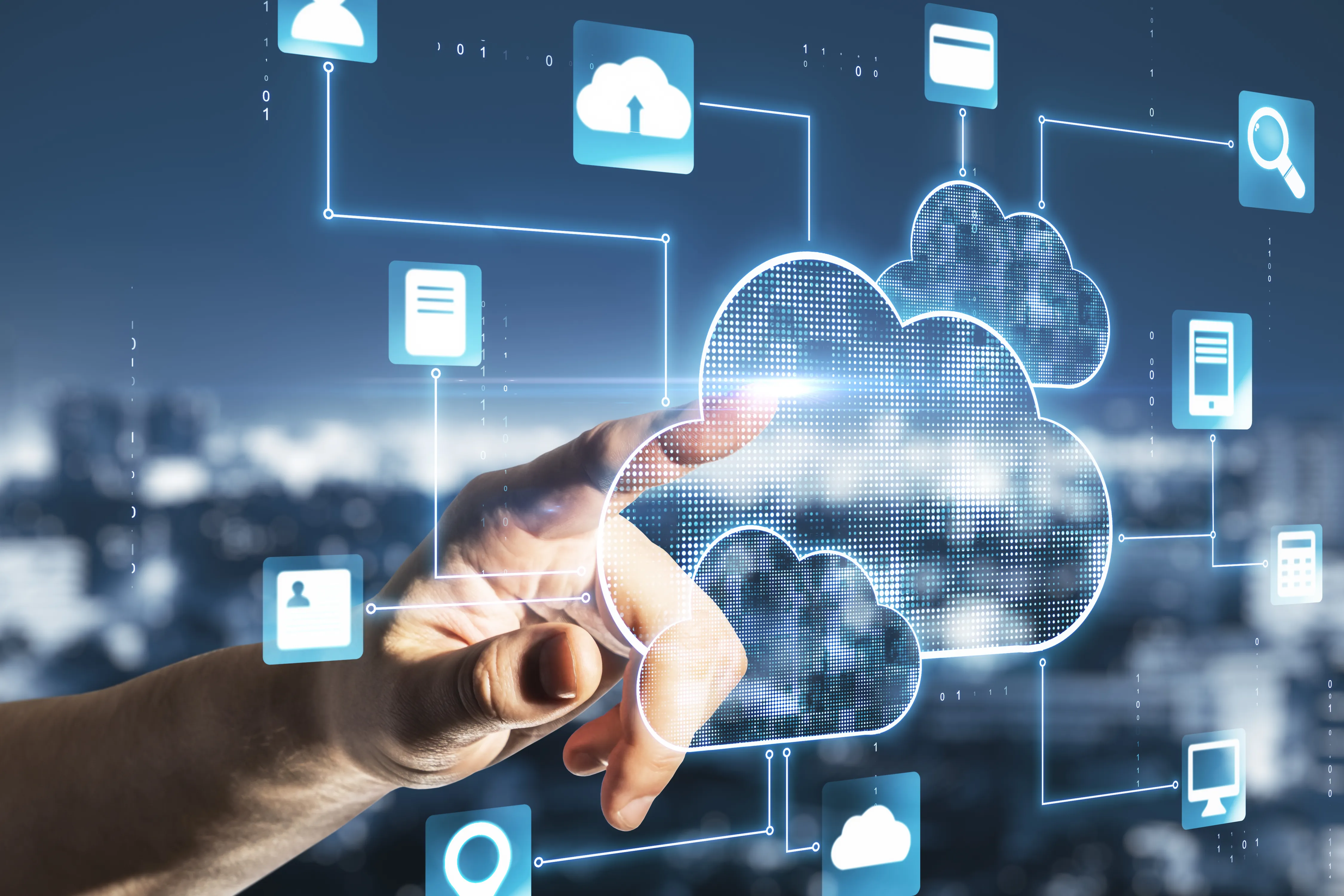
The Impact of Machine Learning and AI on Cloud Costs
Machine learning and AI drive up cloud computing costs in various ways. It requires significant data volumes and computing resources to train high-quality AI models. Once trained, data must be stored and models continuously improved to align with rapid advancements.
Resource Intensive Workloads
- AI workloads demand specialized hardware (e.g. GPUs, NPUs), leading to increased costs.
- Fluctuating workloads complicate resource planning and budgeting.
Solutions Offered by AI and Machine Learning
The good news? AI and ML also present effective solutions for managing costs.
Optimizing Cloud Resource Use
- AI-powered optimization algorithms dynamically adjust resource levels to match demand.
- Predictive models forecast peak usage to prevent wasteful expenses during low-demand periods.
FinOps and AI Enhancing Cost Management
AI-driven FinOps enables real-time monitoring of cloud costs, providing insights for efficient resource allocation and identifying savings opportunities.
Positioning for Sustainable Success
The integration of FinOps with AI is transformative, providing measurable savings and resource management opportunities. By embracing predictive modeling and real-time monitoring, companies can enhance revenue and optimize operational expenses.
This article was prepared using information from open sources in accordance with the principles of Ethical Policy. The editorial team is not responsible for absolute accuracy, as it relies on data from the sources referenced.